Lasso regression is a type of linear regression that is used for feature selection and regularization in machine learning. It is also known as the Least Absolute Shrinkage and Selection Operator (LASSO). This technique is used to handle multicollinearity, which is when there is high correlation between predictor variables in a regression model. Lasso regression is a powerful tool for building predictive models and can help improve the accuracy and interpretability of the results. Lasso Regression
The term "kitchen sink" is often used in reference to regression analysis to describe a model that includes all possible predictor variables, regardless of their significance or relevance. This approach is also known as "throwing everything in but the kitchen sink". While it may seem like a comprehensive and thorough approach, it can actually lead to overfitting and a lack of interpretability in the results. Kitchen Sink
Regression analysis is a statistical technique used to explore the relationship between a dependent variable and one or more independent variables. It is commonly used in data analysis and predictive modeling to identify patterns and make predictions about future outcomes. There are many different types of regression analysis, including lasso regression and kitchen sink regression, each with their own benefits and drawbacks. Regression Analysis
Feature selection is the process of selecting the most relevant and significant features from a dataset to use in a predictive model. This is important because including irrelevant or redundant features can lead to overfitting and impact the accuracy and interpretability of the results. Lasso regression is a popular technique for feature selection as it can automatically identify and remove irrelevant features. Feature Selection
Machine learning is a subset of artificial intelligence that involves using algorithms and statistical models to enable computers to learn from data and make predictions or decisions without being explicitly programmed. Regression analysis is a commonly used technique in machine learning for supervised learning, where the model is trained on a dataset with known outcomes and then used to make predictions on new data. Lasso regression is a popular technique in machine learning for its ability to handle large datasets and high-dimensional data. Machine Learning
Regularization is a technique used in regression analysis to prevent overfitting and improve the generalizability of the model. It involves adding a penalty term to the model's cost function that penalizes complex models with many predictor variables. Lasso regression uses a type of regularization called L1 regularization, which shrinks the coefficients of irrelevant or redundant features to zero, effectively removing them from the model. Regularization
Multicollinearity is a common issue in regression analysis where there is a high degree of correlation between predictor variables. This can lead to unreliable and unstable estimates of the coefficients, and make it difficult to interpret the results. Lasso regression is a useful technique for handling multicollinearity as it can automatically select the most relevant features and reduce the impact of highly correlated variables. Multicollinearity
Model complexity refers to the number of predictor variables and the interactions between them in a regression model. A complex model with many predictor variables can lead to overfitting and make it difficult to interpret the results. Lasso regression is a useful tool for reducing model complexity by automatically selecting the most relevant features and shrinking the coefficients of irrelevant or redundant variables. Model Complexity
Variable selection is an important step in regression analysis as it involves choosing the most relevant and significant features to include in the model. This is important because including irrelevant or redundant features can lead to overfitting and impact the accuracy and interpretability of the results. Lasso regression is a popular technique for variable selection as it can automatically identify and remove irrelevant features. Variable Selection
Overfitting is a common issue in machine learning where the model performs well on the training data but fails to generalize to new data. This can occur when the model is too complex and captures the random noise or idiosyncrasies in the training data. Lasso regression is a useful technique for preventing overfitting as it reduces the model's complexity and automatically selects the most relevant features. Overfitting
The Battle of Lasso Regression vs Kitchen Sink in House Design
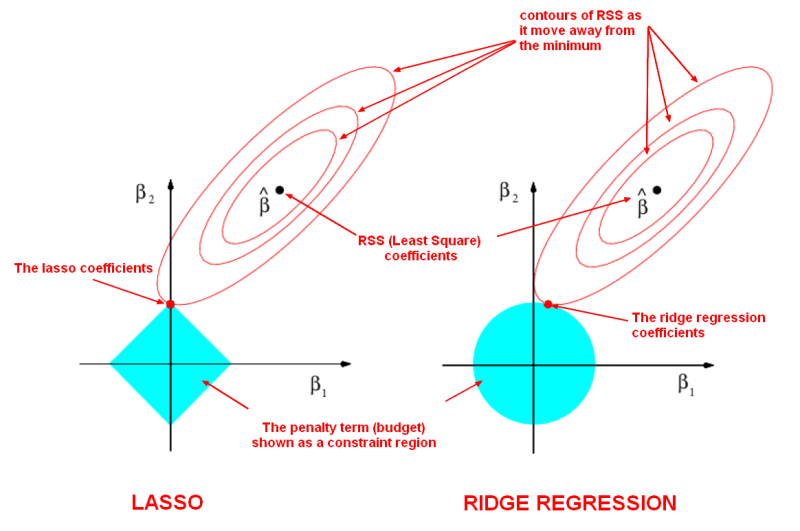
Introduction to House Design

The Basics of Lasso Regression

The All-Inclusive Approach of Kitchen Sink
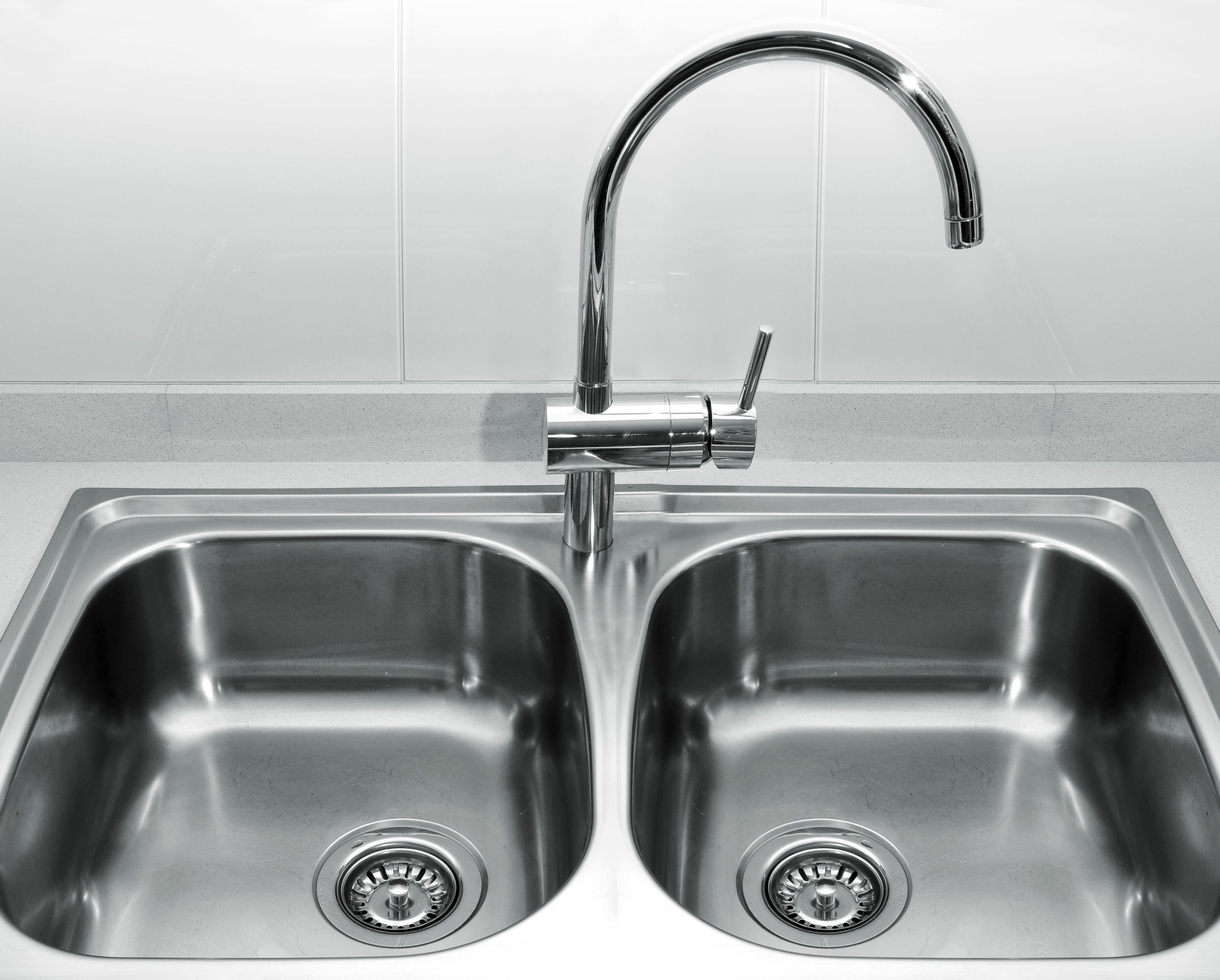
Which One is Better?
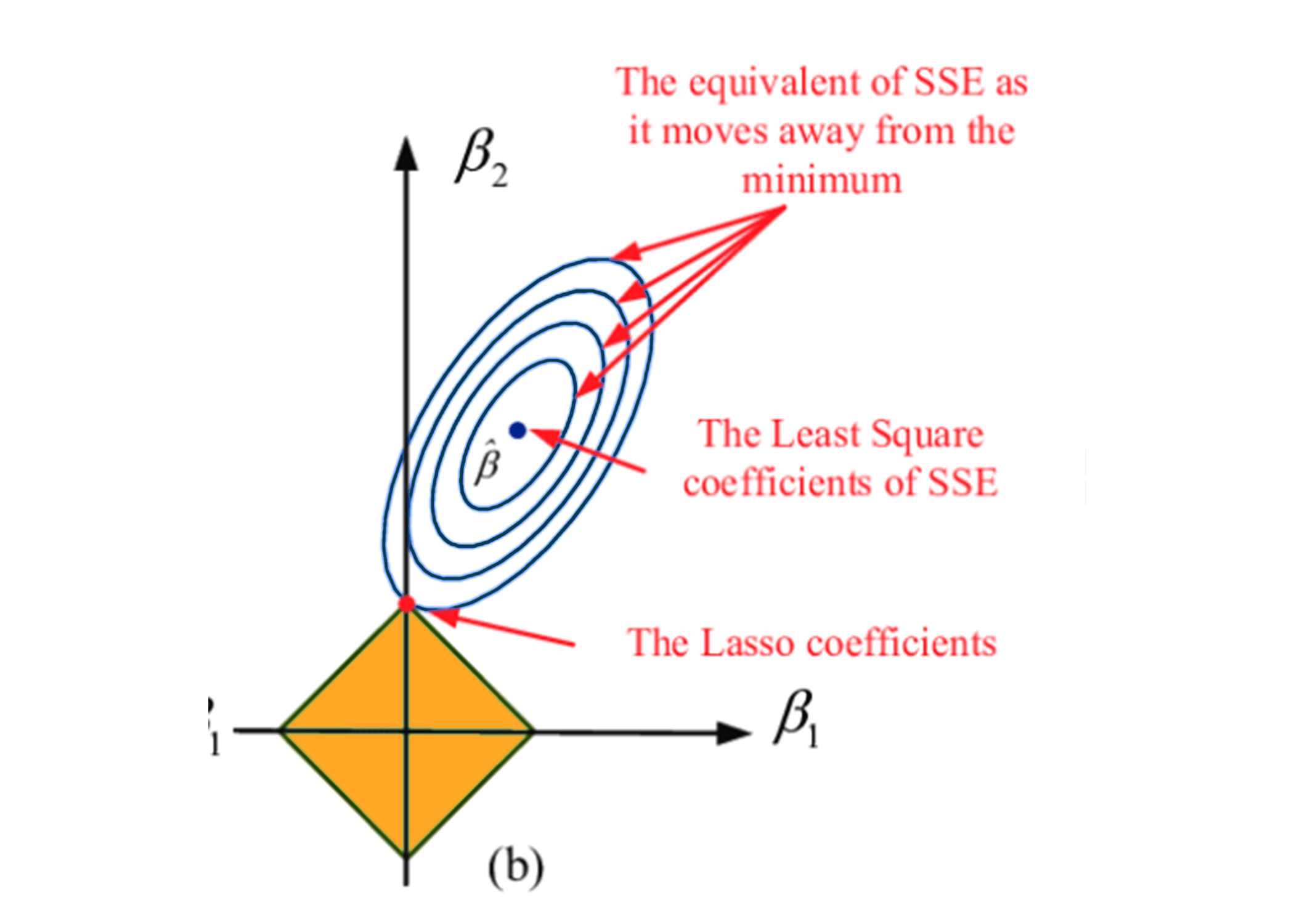
Conclusion
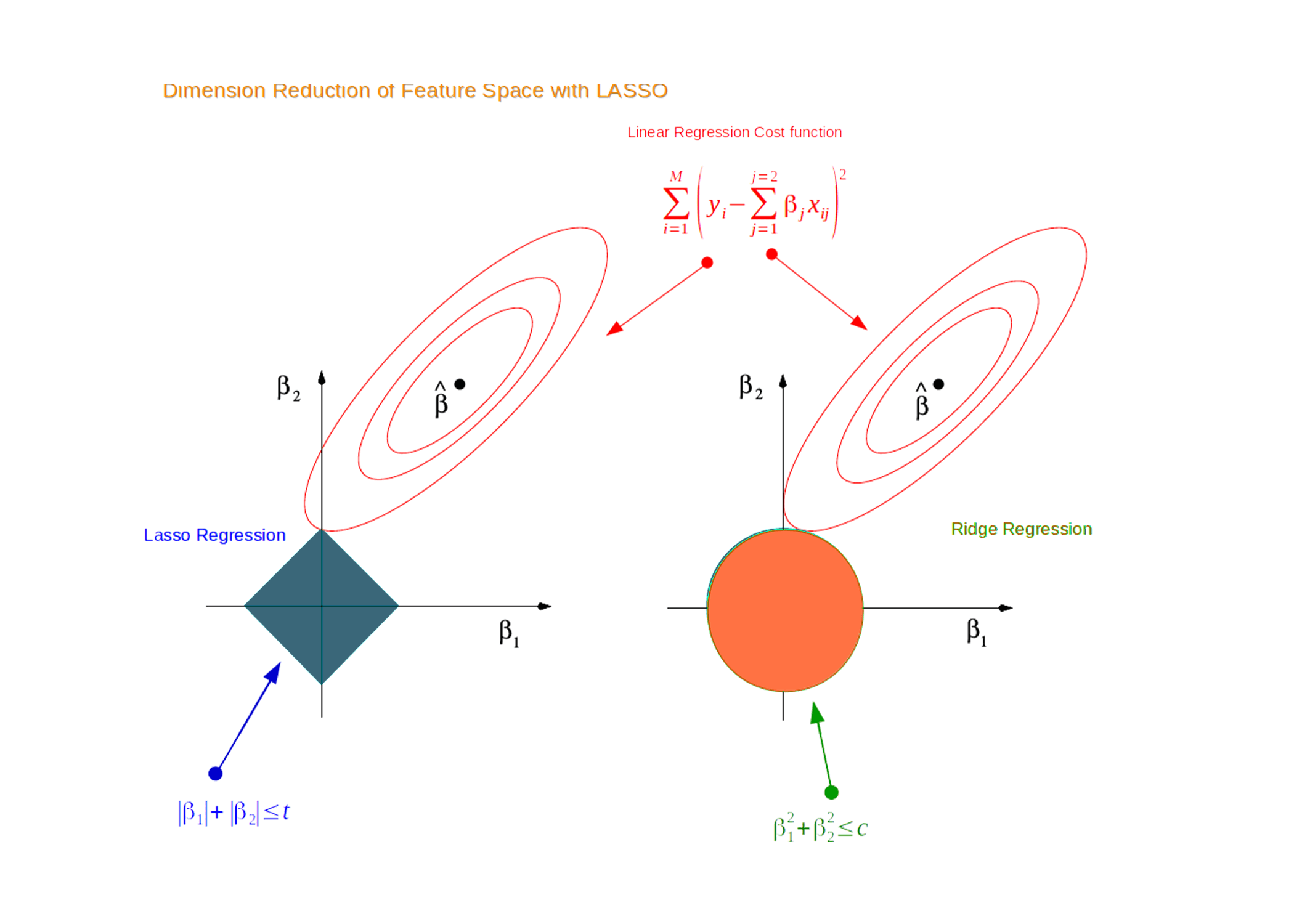